Understanding How CQL Engines Work
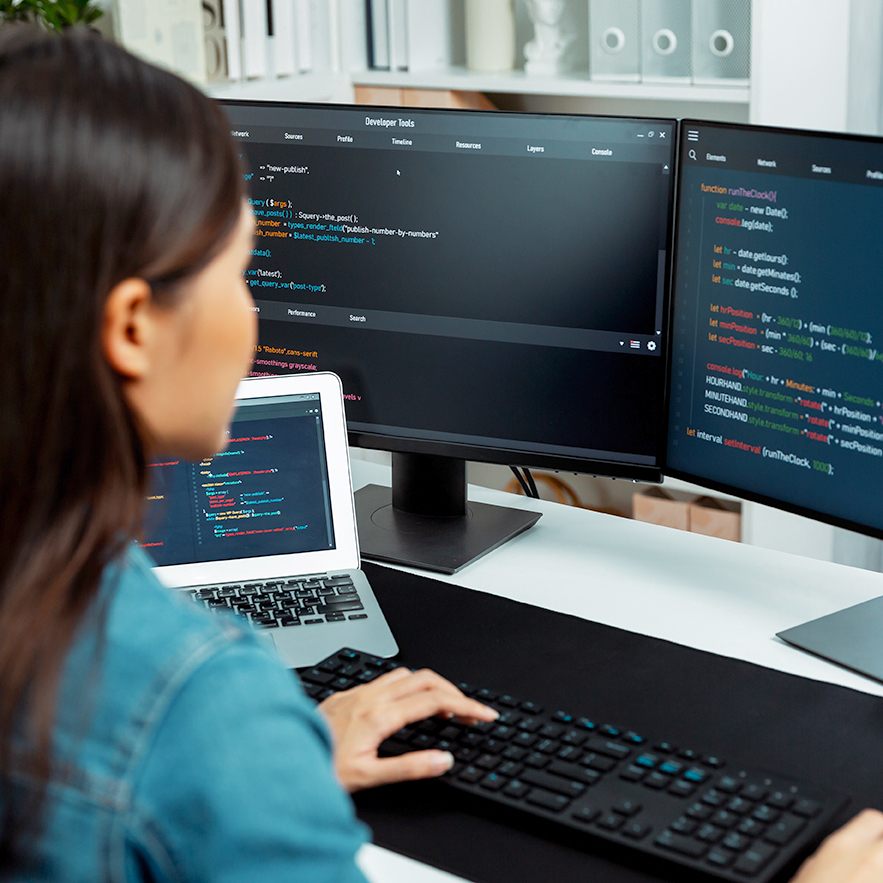
A CQL engine interprets CQL grammar through lexical analysis and parsing of components to identify a measure's population criteria, breaking down CQL statements into tokens and structures that it can understand and process. The engine then uses these parsed components to determine the criteria for including a patient or population in a digital quality measure, such as a HEDIS® measure.
Interpreted CQL logic is integrated with Fast Healthcare Interoperability Resources (FHIR®) queries to import relevant information from EHRs and other health care systems. FHIR provides a standardized format for representing health care data, which aligns with CQL's requirement for consistent and structured data to execute quality measurement logic accurately. Because CQL relies on access to diverse datasets from multiple health care systems, FHIR's standardized approach enables seamless data exchange, allowing CQL engines to retrieve and process data from different sources. Imported data are evaluated against the measure criteria defined in the CQL.
Data from the EHR are parsed and processed based on a measure's defined criteria. A MeasureReport is created for FHIR measures. For eCQM measures created in CQL/QDM (Quality Data Model), the output is based on Clinical Document Architecture. Regardless of the data model used, two types of files can be created.
- An individual MeasureReport in FHIR or a Quality Reporting Document Architecture (QRDA) CAT 1 file for eCQMs. These files contain patient-level data.
- An aggregate MeasureReport in FHIR or a QRDA CAT 3 file for eCQMs. These files contain aggregated results for reporting.
For the MIPS program, QRDA files are submitted by clinicians as part of their quality reporting obligations to regulatory bodies or payers. These reports help assess the quality of care provided, and ensure compliance with health care standards and regulations.
The diagram below depicts how these specifications can be used across different implementation environments.
Source: https://build.fhir.org/ig/HL7/cql/01-introduction.html
This CQL-based solution can be extended to meet Clinical Decision Support (CDS) requirements as well. By using the same technical approach to define CDS logic, the system can deliver real-time alerts and recommendations to clinicians to help them make informed treatment decisions, leading to better patient outcomes. Additionally, integrating CDS with quality reporting tools enables clinicians to improve their performance on quality measures by offering guidance on best practices and evidence-based care. This dual functionality enhances both the quality of care and the efficiency of health care delivery.
Find details on how CQL engines work here.